How to Measure the Data Governance Maturity Model?
Data governance protocols do not exist in an organization, and data quality cannot be guaranteed. When data is unstructured and changes made to it are not documented, its quality degrades rapidly. Not only is this the biggest headache for data teams, but it also hinders business users from innovating with company data. Poor quality data and non-existent data management processes can lead to inaccurate datasets.
When data is wrong, it can have catastrophic consequences, from harmful business decisions to potential data breaches and costly compliance breaches. To address these issues, organizations must deploy a data governance strategy, but for that strategy to be successful, a high level of data maturity is required. The best way to achieve this is to adopt a data governance maturity model.
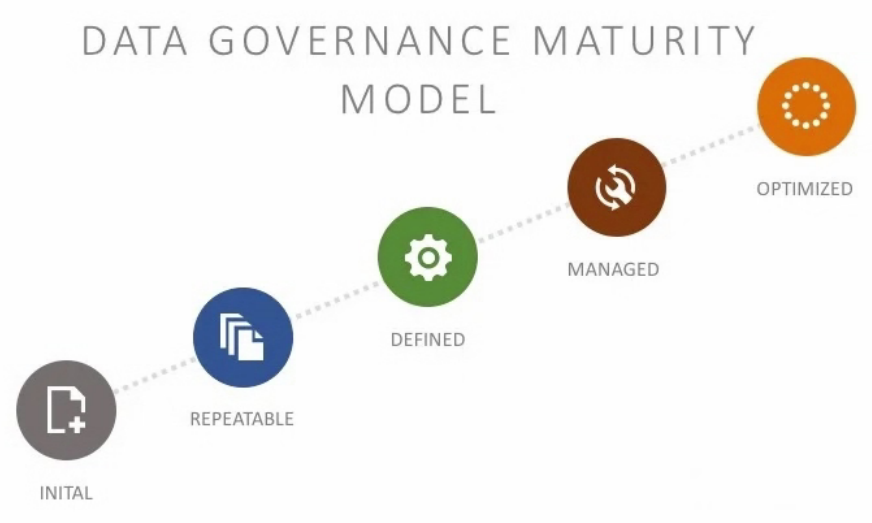
Data Governance Maturity Model
Data Governance Maturity and Its Model
To achieve a higher level of data governance maturity, organizations must adhere to the data governance maturity model. There are many examples of this model, but before we dive into the most well-known examples, let’s understand the terminology.
What exactly is data governance maturity?
Data governance maturity refers to the stage an organization has reached in terms of implementing and adopting a data governance program. An immature organization will have a lot of unorganized data and will not use it to drive growth. Alternatively, mature organizations will be fully aware of the importance of data as a critical business asset and will manage it accordingly.
What is the data governance maturity model?
A data governance maturity model is a tool and methodology for measuring and simply communicating an organization’s data governance program to the entire organization. In mature organizations, all the processes for managing, accessing, and innovating with data assets are in place. Less advanced organizations can use maturity models to achieve this.
There are some well-known data governance maturity models, including examples from IBM, Stanford, Gartner, and Oracle. These models provide a means by which businesses can learn how to effectively manage data, provide user access, ensure high-quality data, and enable everyone in the organization to benefit from these advancements.
There is no one-size-fits-all model for data maturity, and even if one is chosen, it needs to be tuned to fit the organization. When an organization reaches the highest level of data governance maturity, visible results are seen. Across the company, data will be used to innovate and collaborate and make better business decisions, and these organizations will avoid hefty fines for non-compliance with data protection regulations.
Which data governance maturity model should be used?
Although there are several data governance maturity models, you need to consider many factors when starting to decide on a data governance maturity model. These include key business drivers, the budget required to implement the model, existing data management and governance frameworks, and your industry.
Progressive Data Governance Maturity Model
The goals of each data governance maturity model are the same, but neither Gartner nor IBM provides the details needed to overcome the challenges businesses will face. Organizations are able to track the progress of their data governance programs.
Level 1: undefined
- Not knowing the importance of data;
- No action;
- Flow is reactive and often chaotic;
Level 2: aware
- Realize the importance of data;
- Existing data practices are understood and documented;
- Provide a list of data sources;
Level 3: defined
- Data governance rules and policies are defined;
- Identify data owners and data stewards;
- Governance committee established;
- Installed data directory;
Level 4: implemented
- Enforce data governance policies and enforcement rules;
- Trained;
- Collect and measure data;
- Set up alerts to monitor data quality issues raised by users;
Level 5: optimization
- Optimized rules and policies to improve efficiency;
- Redesigned workflow reduces redundancy;
- Data is flagged by users for increased discoverability;
The data governance maturity model should be applied to the three core areas of data governance: data quality, data access management, and data literacy. The aim is to apply the model independently to each of the three domains and gradually address the data governance problem.
The best way to understand where your organization is and move to the next level is to ask data users questions through a formal questionnaire. This will be able to understand where the organization is, what employees know, and what they don’t know.
IBM Data Governance Maturity Model
The IBM Data Governance Maturity Model is one of the most widely known. The model was developed in 2007 to help organizations determine progress in 11 core data governance areas. These include data awareness and organizational structure, data policy, data governance, data quality management, data lifecycle management, IT security and privacy, data architecture, data classification, compliance, value creation and auditing.
Level 1: initial
- Limited to no data processes or governance;
- Data management is ad hoc and reactive;
- No formal procedures for tracking data;
- Missing deadlines and exceeding project budgets;
To upgrade to Level 2, data teams should review how data is shared across their organization and develop a plan that includes data owners and other stakeholders.
Level 2: managed
- Users realize the business value of data;
- Some data projects, such as map data infrastructure, are in progress;
- Little automation;
- Data governance measures agreed and available for use;
- Data teams start focusing on metadata;
To reach Level 3, regulatory measures need to be further developed and documented. To do this, start creating models that map critical infrastructure and requirements.
Level 3: defined
- Data policy is clearly defined;
- A number of data stewards have been identified and appointed;
- There are some data management techniques in use;
- Data integration plans are being developed;
- Users are sharing and understanding data management processes;
- Master data management is commonplace;
- Data quality risk assessment measures are being used;
Organizations will move to Level 4 as they continue to specify and implement data policies and governance processes.
Level 4: quantitative management
- Data policy is clearly defined;
- Enterprise-level data governance measures in place;
- Clear data quality goals in place;
- Data models are readily available;
- Data governance principles drive all data projects;
- Performance management is live and in progress;
To achieve the highest level of data maturity, you must focus on generating KPIs and other performance metrics. To do this, a clear, concise plan for implementing the data model must be in place.
Level 5: optimization
- Data management cost reduction;
- Automation is common;
- Company-wide adoption of clear and comprehensive data management principles;
- Data governance is part of company culture;
- Calculating and tracking the ROI of data projects is standard practice;
A mature organization will fully appreciate the importance of data as a critical business asset and will manage it accordingly.
Gartner Data Governance Maturity Model
Another widely recognized model is the Gartner Data Governance Maturity Model. Since 2008, the Gartner model has enabled organizations to achieve five primary goals:
- Company-wide data integration;
- Unified content;
- Master data domain integration;
- Smooth information channels;
- Metadata management;
Level 0: undefined
- No data governance, data ownership or accountability;
- No process or structure for information sharing;
- No standardization or metadata management;
- Most archiving and document sharing is done via email;
- No unification, data fragmentation;
- Important business decisions are made with insufficient information;
Action items: Data teams and planners must educate key business leaders about the importance of data governance and focus on the potential impact of violating compliance regulations.
Level 1: awareness
- Absence of data owners is evident;
- Business leaders admit lack of support for enterprise information management (EIM);
- The value of data is becoming more and more obvious;
- Some level of awareness of data quality issues;
- People realize the need for standardized data policies and processes;
- Be aware of redundant reporting and inefficient BI processes;
- The risks of not having an EIM are becoming more apparent;
Action item: The data team must develop an EIM strategy that aligns with the existing enterprise architecture and strategic business goals.
Level 2: reactivity
- Organizations understand the value of corporate data;
- Data begins to be shared across departments, projects and systems;
- The data quality process is reactive;
- Policy in place, but low adoption;
- Data information and retention assessment process under development;
Action items: Key business leaders must facilitate initial procedures and encourage adoption. At the same time, an overall value proposition must be provided.
Level 3: active
- Data managers and owners are identified and active;
- Collaboration is considered a key enterprise process;
- Roles and governance models confirmed;
- Company-wide compliance with governance agreements;
- Data governance is an integral part of the development and deployment of every project;
- Operational risk reduction;
Action items: Create and present an EIM strategy to business stakeholders and management, and identify EIM opportunities at the departmental level.
Level 4: administration
- Enterprise-wide consensus that data is critical;
- Data policy is developed, launched and well understood;
- Data governance body created;
- Data metrics are well defined and easily accessible;
Action item: IT management tasks must be inventoried to check that they comply with EIM policies. There should be a scorecard to evaluate the data management process.
Level 5: effective
- Leveraging data and management information is seen as providing a competitive advantage;
- Has Service Level Agreement (SLA);
- Achieving productivity goals and reducing risk are two goals associated with an EIM strategy;
- The team responsible for EIM is mature and active;
- Core EIM goals achieved;
Action item: Ensure steps are taken to ensure EIM controls and quality standards continue in the event of a leadership change.
When a company reaches the highest level of data governance maturity, visible results are seen.
Data is the most important driver of modern business growth. Not only does it support critical business decisions, but it enables collaborative practices to aid organization-wide innovation.
Conclusion
Thank you for reading our article and we hope it can help you to have a better understanding of data governance maturity model. If you want to learn more about data governance, we would like to advise you to visit Gudu SQLFlow for more information.
As one of the best data lineage tools available on the market today, Gudu SQLFlow can not only analyze SQL script files, obtain data lineage, and perform visual display, but also allow users to provide data lineage in CSV format and perform visual display. (Published by Ryan on Jun 23, 2022)
If you enjoy reading this, then, please explore our other articles below: