How to Improve Data Quality?
Improving data quality can bring us lots of benefits. For example, improving data quality delivers trusted reporting and analysis, optimized operational processes, superior customer experience, and higher ROI. So, we need high quality data and improve data quality. But how to improve data quality effectively? If you are looking answer to this question, then you’ve come to the right place. In this article, we’ll introduce 10 tips on how to improve data quality.
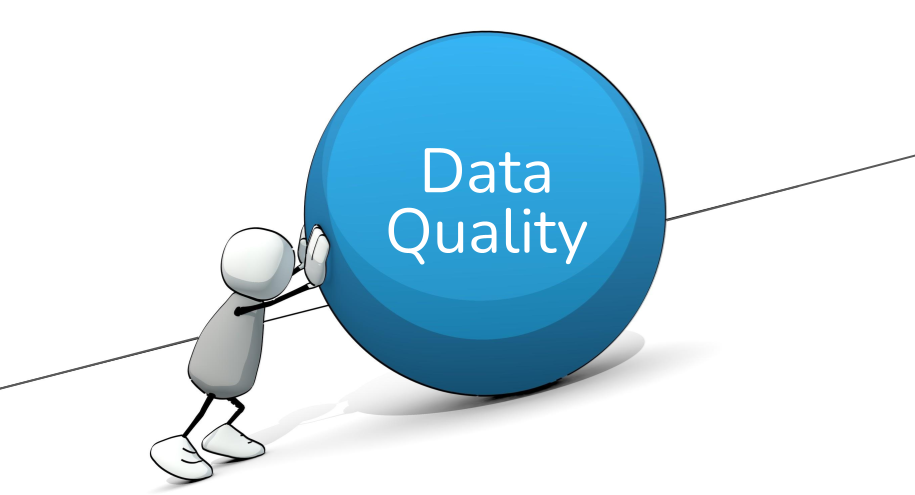
How to Improve Data Quality?
Before diving into our article, let’s figure out what’s data quality.
What’s data quality?
According to Wikipedia, data quality refers to the qualitative or quantitative pieces of information. There are many definitions of data quality, but data is generally considered high-quality if it is “fit for the intended use in operations, decision-making, and planning.” Furthermore, data is considered high quality if it correctly represents the real-world structure it refers to. In addition to these definitions, as the number of data sources increases, the issue of internal data consistency becomes important, regardless of suitability for any particular external purpose.
Views on data quality are often divided, even when discussing the same set of data used for the same purpose. In this context, data governance is used to form agreed data quality definitions and standards. In such cases, data cleansing, including standardization, may be required to ensure data quality.
How to improve data quality?
Following these 10 tips can help you start your long-term journey to better data quality.
- Define business requirements and assess business impact: Generally speaking, our business requirements are the driving force behind our data quality improvement initiatives. Therefore, you can prioritize data quality issues based on your business requirements and their long-term influence on your business. Measuring business influence helps establish goals and track progress in data quality improvement. Ongoing reference to business requirements lays the foundation for an improved data quality approach.
- Understand your data: To fully understand your data, you need to figure out the following questions: Where does it come from, what does it describe, and how can you extract the most value from it? Data intelligence is the ability to properly comprehend and employ data. The best strategic method to improve data quality is to properly describe and connect data throughout the process.
- Resolve data quality issues at the source: Usually, the purpose of temporarily fixing data quality issues is just to continue working. Just imagine what happens if a data scientist finds empty records in a selected dataset. Most likely, she or he will fix the errors in the copy and continue the analytical work. However, if the corrections do not reach the source, the original dataset still has quality issues that affect its subsequent use. Therefore, it can be seen that prevention is better than cure. In this case, we can improve data quality by preventing the propagation of erroneous data.
- Use option sets and normalize your data: Users can make various forms of errors, especially spelling errors, when they enter data in different forms. For example, they might misspell “road” as “roda” and forget it. But when you select these values for analysis, these errors can seriously affect the quality of the dataset. Then how to solve this problem? We can use a defined list of values or option sets for these fields whenever it is possible, so that the user doesn’t make any mistakes. In other cases, the use of normalization tools and techniques can resolve data inconsistencies, thereby improving the quality of the data.
- Promote a data-driven culture: An organization-wide data-driven culture follows a specific set of values, behaviors, and norms that ensure the effective and efficient use of data. Of course, it also requires everyone to fully acknowledge their important role in data quality. Develop a shared organization-wide definition of data quality, identify your specific quality metrics, ensure ongoing measurement of the defined metrics, and plan for error resolution. Additionally, your organization can use data governance to standardize the management of data assets and improve their quality. A key Gartner recommendation is to enable business users to flag and resolve quality issues. With self-service data quality, one can further empower data analysts, data scientists, and business users to identify and solve quality issues on their own. In conclusion, a strong data-driven culture encourages everyone to make their due contribution to data quality.
- Specify a data steward: We can also manage data quality by appointing data stewards. Data stewards can be responsible for analyzing the current state of data quality, optimizing the review process, and implementing the required tools. At the same time, it is their responsibility to oversee data governance and manage metadata. In short, having a data steward in the organization ensures clear accountability and complete oversight for improving data quality.
- Empower your team with DataOps: The DataOps methodology focuses on process-oriented automation and best practices to improve the quality and agility of data analysis. DataOps enables data activation to achieve business value across all technology tiers, from infrastructure to experience. We can innovate DataOps to add automation to the human actions of defining data quality, testing data quality, and fixing data quality failures. Providing a DataOps culture to all teams is a strategic approach to improving data quality.
- Focus on training and reminding:A data-driven culture ensures that the entire organization is involved in data quality. However, it is also important to maintain their interest and contributions through innovative ideas. Moreover, regular training in concepts, metrics and tool usage will help to reinforce the needs and benefits of data quality. Sharing quality issues and success stories across the organization can serve as a friendly reminder. In addition, providing professional training to employees is an effective way to improve data quality.
- Prevent future data errors: Data quality is not only about correcting current mistakes, but also about preventing future ones. The key is to assess and address the root cause of data quality issues in your organization. Are these processes manual or automated, are the measurements defined correctly, are errors directly correctable by stakeholders, and is a data quality culture firmly in place? The data quality solution that you choose should focus on enabling data quality across your organization.
- Communicate actions and results: It’s highly important to get everyone involved in data quality projects because today’s data quality isn’t limited to a handful of teams. Making all stakeholders aware of these activities can generate interest and foster engagement. If you communicate frequently about data quality errors, possible causes, plans, tests, and results, more people will be actively involved in improvement projects. Documenting progress, actions and results further increases the organizational knowledge base to drive future planning.
Conclusion
Thank you for reading our article and we hope it can help you to have a better understanding of how to improve data quality. If you want to learn more about data quality, data governance, data stewards, data analysts, data scientists and data lineage, we would like to advise you to visit Gudu SQLFlow official website for more information.
As one of the best data lineage tools, Gudu SQLFlow can not only analyze SQL script files, obtain data lineage, and perform visual display, but also allow users to provide data lineage in CSV format and perform visual display. (Published by Ryan on May 20, 2022)
6 Comments
Leave A Comment
If you enjoy reading this, then, please explore our other articles below:
[…] data or even erroneous data will inevitably “tell lies”! Data governance is to improve the quality of data and give full play to the value of data […]
[…] is an important part of an enterprise’s development strategy. It is a plan to maintain and improve data quality, integrity, security, and access, and it is the highest principle that guides data governance. […]
[…] At the same time, problems in the area of data quality can be identified. When we find that different users use the same data set to analyze different results, it helps to identify personnel training problems and areas that need to improve data quality. […]
[…] operation process. Although technology-driven data governance can also reveal data deficiencies and improve data quality, management and business personnel seem not satisfied with […]
[…] can be simplified by the application, such as creating data dictionaries, discovering and fixing data quality issues, and obtaining information on historical data changes, but this is not data […]
[…] it is necessary to preprocess the data so that the data can be used by us. Data preprocessing can improve data quality, including accuracy, completeness, and consistency. The methods of data preprocessing include data […]