15 Best Practices for Data Governance
In this post, we’ll introduce 15 best practices for data governance. Before diving into our article, let’s figure out what’s data governance. Data governance is a set of principles and practices to ensure the quality of data throughout its life cycle. The Data Governance Institute (DGI) believes it is a set of practical frameworks to help the various data stakeholders of any organization identify and meet their information needs. DGI believes that companies need not only systems for managing data but also a complete set of rules, with processes and procedures to ensure that these rules are followed. This is a daunting task for any governance system.
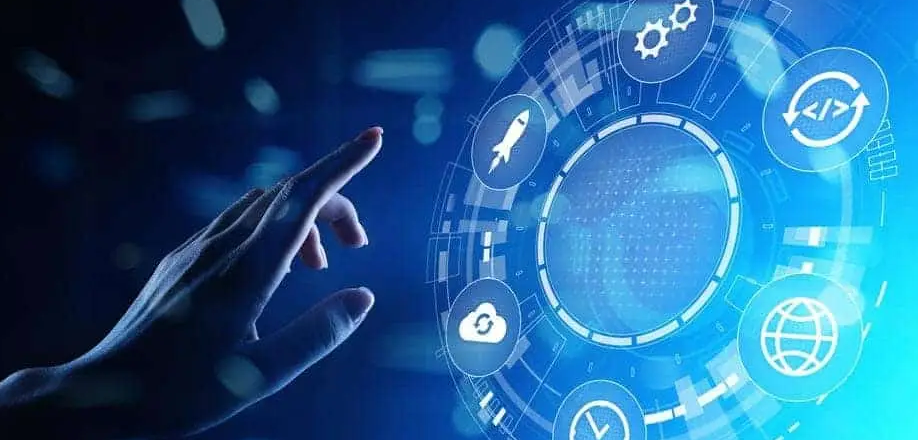
Best Practices for Data Governance
Data is becoming a core business asset that determines business success. Digital transformation is on the agenda all over the world. If you can manage your data, you can leverage data assets and successfully digitally transform. This means deploying a data governance framework that fits the organization’s growth, future business goals, and business model. The framework must control the data standards required in this process and delegate the required roles and responsibilities within the organization and within the business ecosystem in which the company operates.
15 Best Practices for Data Governance
On the one hand, you can learn from other processes engaged in data governance. However, every organization is different, and you need to adjust your data governance practices along the way from the unconscious maturity stage to the effective maturity stage. Here are 15 best practices for data governance:
- Start small. In all aspects of business, don’t be arrogant. Aim to win quickly and build ambition over time.
- Set clear, measurable, and specific goals. You can’t control what you can’t measure. Celebrate when you reach your goal and use it to win the next one.
- Define ownership. Without enterprise ownership, a data governance framework cannot succeed.
- Identify relevant roles and responsibilities. Data governance is a team effort that includes deliverables from all parts of the business.
- Educate the stakeholders. Use business terminology whenever possible and translate the academic parts of the data governance discipline into something meaningful in a business context.
- Focus on the business model. A data governance framework must be integrated into the way business is conducted in an enterprise.
- Map infrastructure, architecture and tools. Your data governance framework must be a logical part of the enterprise architecture, IT environment, and required tools.
- Develop standardized data definitions. It is necessary to distinguish what needs to be managed centrally and what needs to be managed agile and localized, and strike a balance between the two.
- Identify data fields. Start with the data domain to get the best ratio between impact and action to increase data governance maturity.
- Identify key data elements. Focus on the most critical data elements.
- Define control metrics. Deploy these in the most meaningful business processes, IT applications and/or reports.
- Build a business case. Identify the benefits of increasing data governance maturity related to growth, cost savings, risk, and compliance.
- Use metrics. Focus on limited data quality KPIs related to general corporate performance.
- Communicate frequently. Data governance practitioners believe that communication is the most critical part of the discipline.
- This is a practice, not a project.
How is data governance implemented?
The field of data governance includes but is not limited to data standards, data quality, metadata, data models, data distribution, data storage, data exchange, data life cycle, data security, etc. The field of data governance changes with the development of banking business, and the relationship between the fields also needs to be continuously excavated and analyzed, and finally a field network of mutual coordination and verification will be formed to improve the effectiveness of data governance in an all-round way.
- Data architecture management: planning and managing the logical framework for the distribution, transmission and storage of data from the generator to the consumer;
- Data model management: the information model of an enterprise is a graphical representation of enterprise data standards;
- Data standard management: standardize the data record format of important activities and objects of the enterprise;
- Data quality management: continuous monitoring and evaluation of the standardization, accuracy, consistency, integrity and timeliness of data;
- Metadata management: registering enterprise data assets and recording their relevance;
- Data security management: setting security levels for data to ensure that it is used appropriately;
- Master data management: the process of uniformly defining, centrally saving, publishing, updating and deleting business data that is critical to the enterprise and shared across systems;
- Data life cycle management: manages the entire process of data generation, storage, transmission, use and destruction.
Conclusion
Thank you for reading our article and we hope it can help you to have a better understanding of the best practices for data governance. If you want to learn more about data governance, we would like to advise you to visit Gudu SQLFlow for more information.
As one of the best data lineage tools available on the market today, Gudu SQLFlow can not only analyze SQL script files, obtain data lineage, and perform visual display, but also allow users to provide data lineage in CSV format and perform visual display. (Published by Ryan on Aug 29, 2022)
If you enjoy reading this, then, please explore our other articles below: