Data Governance Framework: The Cornerstone of a Data-driven Enterprise
In a data governance project, formulating a data governance structure is the core task. A good data governance structure can ensure the integrity of data governance, achieve thorough and complete data governance, and better achieve the expected results of data governance. Before diving into our article, let’s figure out what’s data governance framework.
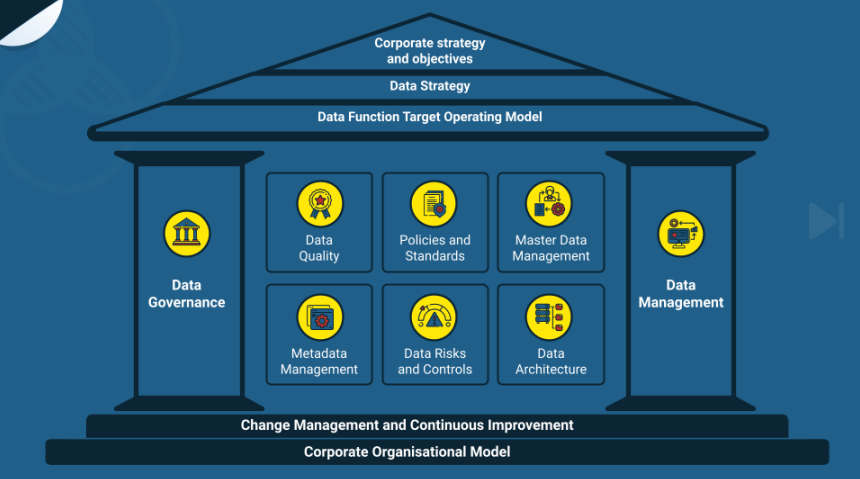
Data Governance Framework
What is a data governance framework?
To explain the data governance framework, we must first define data governance.
- Data governance refers to how an organization leverages its people, processes, and technology to manage its internal data.
- A data governance framework is a specific set of principles and processes that define how data is collected, stored, and used within an organization.
With the right framework in place, organizations can turn their data into valuable, powerful assets that can be used to meet or exceed business goals and objectives.
At a minimum, a data governance framework should establish the following policies for each data asset in the organization:
- Schema: defines how data is organized, retrieved, and stored.
- Usage: specify which employees and third parties have access to the data.
- Privacy: establish usage parameters and limits to minimize legal risk to ensure consumer data privacy and regulatory compliance.
- Categorize: categorize data into sensitivity levels, such as Internal vs. Public or Classified vs. Restricted.
- Standards: establish standards to ensure accuracy, validity, and reliability so that data can be used for decision-making.
10 Core Elements of a Data Governance Framework
People, processes, and technology must be aligned for a data governance program to be successful. Creating the right framework to execute the plan is also important and requires collaboration between dedicated IT professionals, business unit leaders, and data stakeholders. Here is what should be included in a data governance framework.
- Data Strategy: Creating a data strategy is a critical first step in establishing a data governance framework. A data strategy refers to an organization’s high-level operational standards and requirements around data, often reflecting the culture within the organization.
- Data Management: Establishing key processes for data governance is critical to every data governance framework. Typical procedures involve data sharing, data quality monitoring and testing, data issue tracking, etc.
- Data System: The framework also establishes a data governance system for enterprise data. Examples of data regimes include internal and external data sharing, regulatory compliance, data storage requirements, data quality, etc.
- Data Standard: Data standards define an overall approach to ensuring compliance with data policies. Examples of data standards include data modeling standards, naming and abbreviation standards, metadata management, and the like. The most common scenario for data standards is marketing taxonomies – creating a common blueprint of marketing data (campaigns, assets, tags) that every stakeholder can access.
- Data Security: Data security refers to protecting corporate data throughout its lifecycle. The framework should detail the processes for securing data from cyberattacks, unauthorized access, data breaches and theft. It should also have a clear plan of action to deal with all potential threats.
- Data Quality: What’s wrong with low quality data? Data quality determines whether a dataset is fit for its business purpose. Therefore, ensuring high data quality should be a top priority when designing a data governance framework. Establishing policies that prohibit manual entry, require open standards, and restrict data access are good places to start. This is probably the most important component of a data governance framework, as poor data quality can stifle success.
- Communication and Collaboration: Data governance is a strategic initiative that requires an enterprise-wide effort. Therefore, it is critical to develop a plan to communicate the actions and achievements of the data governance team. Clearly define goals, objectives, and tools to foster enthusiastic support and widespread adoption of data governance frameworks. Just like data quality, an internal culture around data sharing, communication, and collaboration can make or break data-driven initiatives and the data strategy itself! This highlights the importance of data literacy.
- Data Literacy: Sometimes a major cultural shift is required for data governance to be successful. This can be achieved by educating or promoting data literacy. Prioritizing data democratization or ensuring that all users can access, understand and utilize data is part of the educational process. Educated users can make better decisions and maximize the value of enterprise data.
- Key Performance Indicators: A data governance program requires a collaborative investment in people and money, so establishing KPIs to measure its business impact is critical. Additionally, KPIs should be measurable and consistently tracked over time to provide stakeholders with an accurate return on investment (ROI).
- Technology: Selecting data governance tools for unique needs is critical to an organization’s data governance strategy. Solutions and integrated data platforms on the market offer a wide range of capabilities, so the needs of the enterprise need to be identified. Most data governance software platforms provide essentials such as creating business glossaries, rules-based workflows, and automation. Additionally, many offer a variety of data capabilities, including data discovery, management, cleansing, and integration.
Why do you need a data governance framework?
The ability to leverage, store, and interpret data has turned information into a valuable asset, enabling organizations to become more agile and competitive in a global business environment. However, this capability comes with a huge responsibility – ensuring that stored data is protected.
While laws and regulations have improved overall data security and compliance, breaches that expose consumers’ personal information continue to make daily news. This has prompted companies to take additional strategic steps to protect their data. By implementing a data governance program, organizations can improve security and realize other valuable business benefits.
Here are the main benefits of effective data governance:
- Better Decision Support: Ensure data is consistent and high-quality across the organization, instilling confidence and generating better insights to support decision-making.
- Reduce Risk: Promote compliance and reduce risks that can threaten the longevity of your business and data security.
- Improved Scalability: Seamless growth is possible no matter how exponentially the amount of data grows.
- Improved Efficiency:Establish transparent processes and policies for data strategy and management, making businesses and their IT departments more agile and scalable.
- Cut Costs: Centrally control data and reduce costs associated with fragmented or siloed data management.
- Enhance Collaboration: Promote a culture of collaboration by providing consistent, accurate data across the organization.
Which businesses benefit most from data governance?
Of course, a well-executed, comprehensive data governance policy benefits the entire enterprise. But they also provide specific benefits for the functional areas of the business.
- Financials: High-quality, timely data provides shareholders with accurate financial reports.
- Marketing Operations: Customer data, engagement rates, lead metrics, and other key marketing data governance metrics support and inform sales and marketing strategies.
- Laws and Regulations: Policies and procedures ensure compliance and consumer privacy, reduce risk and protect the business.
- Efficiency: Governance tools and processes can automate and improve operational efficiency.
- Leadership: Consistent, accurate, high-quality data provides oversight, informs strategy, and identifies potential market opportunities.
- Customer Support: Accurate, complete and centralized data enables customer service representatives to quickly resolve issues and concerns with fewer touchpoints.
Three Data Governance Operating Models
When designing a data governance program, you need to consider which operating model is best for the size and architecture of the business. Models should detail operational guidelines, establish oversight, and provide the infrastructure for data ownership and decision-making. Organizations need to evaluate three basic models: centralized, decentralized, and hybrid.
1. Centralized
In a centralized data governance model, one person is often designated as the data governance lead, responsible for making decisions and providing direction to the project. In some organizations, this person may also be responsible for managing master data and distributing it to users as needed or requested. Other organizations may distribute components decentrally, assigning team leaders to develop datasets and distribute information to their users.
Pros:
- Better Focus: Instead of collecting, managing, and distributing data, teams can focus on essential tasks.
- Quality Control: When data is consistent across the organization, teams can make better decisions for the business.
- Increased Security: Data is easier to track and protect, reducing risks associated with data mishandling.
- Cost Control: Centralizing data requires fewer resources and simplifies processes.
Cons:
- As a top-down approach, a centralized model fosters bureaucracy and fails to meet the unique needs of each area of the business.
2. Decentralized
In a decentralized data governance model, committees typically design and manage enterprise data governance policies. However, the functional parts of the business create and manage their own datasets and handle distributing the information to users.
Pros:
- Improved Representation: Consider all areas of business and their unique challenges.
- Better Data: Data is created by the people who will use it to get work done.
- Increased Efficiency: Relatively easy to set up and more adaptable to changing business needs and requirements.
- Shared Maintenance: Multiple data owners share responsibility for managing and enforcing data governance policies.
Cons:
- Decentralized models can lead to inconsistencies and duplication of master data, affecting consensus, reporting, and decision-making.
3. Federated
In a federated model, a centralized enterprise data governance structure provides the framework, technology, and best practices to follow, but application owners operate autonomously. In other words, each area of the business has its own data and metadata and is free to develop standards, policies and procedures that best suit its business needs.
Pros:
- More Autonomy: teams can manage and scale their own data without affecting other teams;
- Faster Problem Resolution: Problems are resolved when and where they occur;
- Increased Agility: Teams can experiment and adjust data as soon as business needs arise
Cons:
- It is necessary to have deep expertise in each business area to operate autonomously. And additional steps must be taken to ensure data consistency and coordination across teams across the organization.
Conclusion
Thank you for reading our article and we hope it can help you to have a better understanding of the data governance framework. If you want to learn more about data governance, we would like to advise you to visit Gudu SQLFlow for more information.
As one of the best data lineage tools available on the market today, Gudu SQLFlow can not only analyze SQL script files, obtain data lineage, and perform visual display, but also allow users to provide data lineage in CSV format and perform visual display. (Published by Ryan on Aug 20, 2022)
One Comment
Leave A Comment
If you enjoy reading this, then, please explore our other articles below:
[…] data, you can leverage data assets and successfully digitally transform. This means deploying a data governance framework that fits the organization’s growth, future business goals, and business model. The framework […]