Metadata Management for Data Warehouses
Metadata management is the foundation of enterprise data governance and the improvement of data warehouse. As someone who often works with data, the first task is to understand metadata management. This article will sort out the concept of metadata and introduce metadata management for data warehouses.
What is metadata management for data warehouses?
Before going any further, let’s figure out what is metadata and what is a data warehouse.
Metadata, also known as intermediary data and relay data, is data about data. Most of the time, metadata can be divided into business metadata and technical metadata according to the different meanings they represent.
A data warehouse is a central repository of information that can be analyzed to make better decisions. Typically, data regularly flows into a data warehouse from transactional systems, relational databases, and other sources. Business analysts, data engineers, data scientists, and decision makers access data through business intelligence (BI) tools, SQL clients, and other analytical applications.
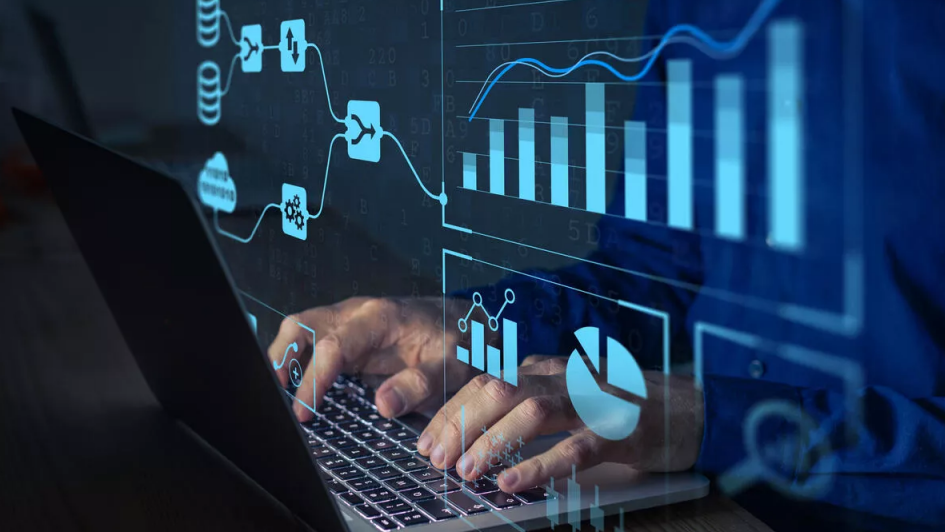
Metadata Management for Data Warehouses
Then what is metadata management for data warehouses?
The metadata in the data warehouse mainly records the definition of each theme, the mapping relationship between different levels, the data status of the monitoring data warehouse and the task running status of the ETL. Generally, metadata is stored and managed uniformly through the metadata repository, and its main purpose is to achieve coordination and consistency in the design, deployment, operation and management of the data warehouse.
Metadata is an important part of data warehouse management system. Metadata management is a key component in enterprise data warehouse. It runs through the whole process of data warehouse construction and directly affects the construction, use and maintenance of data warehouse.
Why do data warehouses need metadata management?
- A must for building data warehouse: The data warehouse is obtained from external data, business data and documents through some ETL tools. Without a clear and clear rule, it is impossible to realize this process.
- Help to quickly understand the data warehouse system: On the one hand, the data warehouse is essentially an important project of a department or even a company, and the development time is lengthy. There will inevitably be a flow of people in the middle. If there is no clear metadata, it will have a major impact on the entire system and the entire project. On the other hand, the data warehouse serves as the analytical data export for the entire department and company, not only for data personnel. The DM layer is unavoidable for business people and DIM for other developers. If there is clear metadata to describe the data warehouse system, it will save a lot of communication time between the two parties.
- Efficient and accurate communication: On the one hand, the management metadata in the metadata records the data permissions of different users, roles, and departments. If there is data that needs to be notified, you can quickly query the system to communicate by mass emails, etc., so as to avoid the situation of lack of people and too many people in the communication link. On the other hand, when communicating with products or communicating with R&D, you can confirm the meaning of indicators and dimensions of mutual communication based on business metadata, so as to avoid the ambiguity of communication at the root, and thus improve the efficiency of communication.
- Guaranteed data quality: The ideal metadata describes the structure of the data warehouse, the schema of the warehouse, the dimensions, measures, hierarchies, definitions of the databases everywhere, and the location and content of the data marts. Therefore, we can judge with certainty which data is definitely accurate, which data may be faulty, and which data is definitely faulty. Simply put, each field should have its value range, business definition and other information. Once metadata is defined, it can be applied to data quality detection, evaluation, etc., so as to truly improve the data quality of enterprises through the data quality management process.
- Reduce data system construction costs: If the metadata is well established, the information will be obtained more accurately and quickly, so that the data system construction will not be reworked or less reworked, the workload of analysis will be reduced, the unified understanding and communication efficiency of all parties will be strengthened, and the development cost will be minimized.
- Quickly analyze change impact: Because metadata is centrally maintained and managed with reference relationships, when changes occur, the metadata management system can be used to analyze in real time the affected business functions, application systems, personnel involved, and whether supervision is involved.
- Prepare for the future: Strategic-level application systems of enterprises such as big data, artificial intelligence, data lake, data center, and business intelligence can rely on good metadata management to exert their due effects.
Metadata Application Scenarios
- Impact analysis: During development, we often encounter the following problems: If I want to change a table or ETL, what will be the impact? If there is no metadata, then we may need to traverse all scripts and data to get the desired answer; however, if there is mature metadata management, then we can get the answer directly and save a lot of time.
- Data lineage analysis: Data lineage analysis is a technical means used to comprehensively track the data processing process, so as to find all related metadata objects starting from a data object and the relationship between these metadata objects. The relationship between metadata objects specifically refers to the data flow input and output relationship representing these metadata objects. After the metadata management system is formed, we can analyze the data health, data distribution, concentration, and data heat in the data warehouse through lineage relationship analysis.
- ETL automation management: In the warehouse, a large part of ETL are boring and repetitive steps. For example, at the source system-ODS layer: table input – table output. Another example is ODS-DW: SQL input – data cleaning – data processing – table output. The above rules are actually part of the metadata. That can be achieved in theory, write a fixed script, and then select it through the front-end – or api interface. In this way, the repeated ETL can be automatically managed to reduce the time cost of ETL development.
- Data quality management: The logic of data cleaning can be simply divided into different data types and designated special processing columns. We only need to specify default cleaning rules for different data types and special processing logic for some special columns to achieve intelligent and fast data cleaning. Data quality management belongs to the intersection of data governance and metadata management, and is more inclined to data governance.
- Data security management: In the data center that Ali advocates, all data interface indicators will be exported from the data warehouse. Therefore, in theory, you only need to configure the metadata management permission in this metadata to achieve data security management for the whole company.
Conclusion
Thank you for reading our article and we hope it can help you to have a better understanding of metadata management for data warehouses. If you want to learn more about metadata management for data warehouses, we would like to advise you to visit Gudu SQLFlow for more information.
As one of the best data lineage tools available on the market today, Gudu SQLFlow can not only analyze SQL script files, obtain data lineage, and perform visual display, but also allow users to provide data lineage in CSV format and perform visual display. (Published by Ryan on Jun 25, 2022)
One Comment
Leave A Comment
If you enjoy reading this, then, please explore our other articles below:
[…] cloud data mining technology, users can retrieve important information from a virtual integrated data warehouse, thereby reducing storage and infrastructure […]