7 Challenges Faced by Data Scientists and How to Solve Them
All professions come up against certain obstacles or challenges, and the role of data scientist is no exception. Many companies fail to take full advantage of data scientists, putting them in the wrong role or not providing the necessary requirements. According to LinkedIn, the top 10 skills for data scientists today include machine learning, big data, data science, R, Python, data mining, data analytics, SQL, MatLab, and statistical modeling. Most data scientists can use these skills in their computers. However, these skills are not sufficient to put them in the right roles to achieve optimal business growth. In this article we’ll explore the common challenges faced by data scientists today.
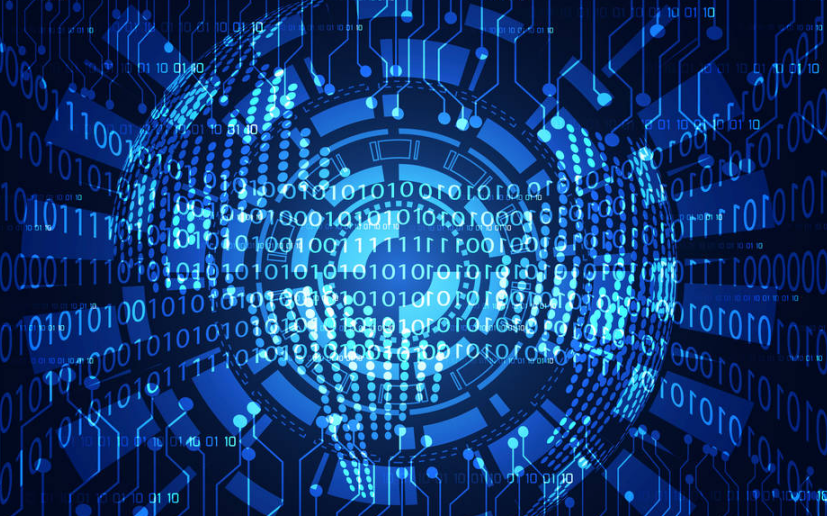
Challenges Faced by Data Scientists
Challenges Faced by Data Scientists – 1. Preparing Data for Intelligent Enterprise AI
The most important function of a data scientist is to identify and prepare the correct data. According to a survey by CrowdFlower, nearly 80% of data scientists are cleaning, organizing, mining, and collecting data from different datasets every day. Here, the data is thoroughly examined before it is analyzed and further worked on. It’s a grueling process, and 76% of data scientists consider it one of the worst parts of their job. The data wars require data scientists to streamline terabytes of data in different formats and codes on different platforms while maintaining logs to prevent duplication of data in the system.
The best way to overcome this is to adopt AI-based technologies that allow data scientists to stay sharp and more powerful in their functionality. Augmented Learning is another versatile enterprise AI tool that can help and assist with data preparation and provide insight into the problem at hand.
Challenges Faced by Data Scientists – 2. Generate Data from Multiple Sources
Organizations obtain data from different applications, software, and tools in a variety of formats. Processing large amounts of data is a huge challenge for data scientists. This process requires manually entering and compiling data, which is time consuming and can lead to duplicate or incorrect decisions. Data can be most useful when it is used appropriately to best function in enterprise AI.
An enterprise can build an intelligent virtual data warehouse with a centralized platform to integrate all data sources in one place. Data from a central repository can be controlled or enhanced to meet and improve enterprise efficiency. This simple fix can save valuable time and effort needed by data scientists.
Challenges Faced by Data Scientists – 3. Identify Business Problems
Problem identification is an important aspect of stable operation. Before building datasets and analyzing data, data scientists should focus on identifying key issues related to business operations. Before setting up a dataset, it is necessary to get to the root of the problem rather than jump to a mechanistic approach.
A data scientist can maintain a regulated workflow before starting any analysis process. The workflow must take into account all business stakeholders and key parties. Special dashboard software provides a range of visual widgets that can be used to make data more meaningful to the enterprise.
Challenges Faced by Data Scientists – 4. Communicate Results to Non-technical Stakeholders
The role of data scientists is aligned with business strategy and their fundamental goal is to improve decision making in the organization. The biggest challenge for data scientists is communicating their results or analysis to corporate executives. Most managers or stakeholders do not understand the tools and equipment used by data scientists, so it is critical to provide them with the right basic ideas in order to implement the model through enterprise AI.
Data scientists need to adopt concepts such as data storytelling to provide a strong narrative for their analysis and visualization of concepts.
Challenges Faced by Data Scientists – 5. Data Security
Rapid upgrades are turning organizations to cloud management to store their important data. Cloud storage is threatened by cyberattacks and online spoofing, leaving confidential data vulnerable to outside attacks. To prevent these cyberattacks, strict regulations have been implemented to protect data in central repositories. The new guidelines force data scientists to bypass these new regulations, making their jobs more complicated.
To overcome threats to security, organizations must install advanced encryption and machine learning security systems to protect data. These systems must comply with all security specifications and are designed to avoid time-consuming audits in order to improve operational efficiency.
Challenges Faced by Data Scientists – 6. Efficient Collaboration
Data scientists often work with data engineers on the same projects for organizations. Then good communication channels are essential to eliminate any conflicts. The organization should take steps to establish good communication channels to ensure that the workflow of both teams matches. Companies can also set up a chief executive to oversee whether the two divisions are working on the same line.
Challenges Faced by Data Scientists – 7. Selection of Non-specific KPI Indicators
There is a misconception that data scientists alone can do most of the work and provide ready-made solutions to all the problems an organization faces. This puts enormous pressure on data scientists and reduces their productivity.
It is essential for every organization to have a set of defined metrics against which to measure the analysis presented by data scientists. In addition, they must examine the impact of these indicators on business operations.
Working as a data scientist is a challenging job because of the variety of tasks and requirements. However, it is one of the most in-demand jobs on the market today. The problems faced by data scientists can be easily reduced to improve the productivity and functionality of enterprise AI in demanding work environments.
Conclusion
Thank you for reading our article and we hope it can help you to have a better understanding of the challenges faced by data scientists. If you want to learn more about data scientists, we would like to advise you to visit Gudu SQLFlow for more information.
As one of the best data lineage tools available on the market today, Gudu SQLFlow can not only analyze SQL script files, obtain data lineage, and perform visual display, but also allow users to provide data lineage in CSV format and perform visual display. (Published by Ryan on Aug 14, 2022)
If you enjoy reading this, then, please explore our other articles below: